As organizations implement AI strategies, they must navigate important questions about copyright and content usage. Understanding how copyright intersects with AI workflows is essential for building effective, compliant systems that can deliver results while respecting intellectual property rights.
The Role of Copyright in the AI Lifecycle
Copyright touches multiple points in the AI lifecycle, often in ways that aren’t obvious. High-quality training material—typically copyrighted content—is essential for developing effective AI models, but even content lawfully acquired through subscriptions is rarely covered by secondary rights for AI activities.
From a technical perspective, modern AI systems don’t merely “read” content like humans do. Despite claims that machines simply process information without making copies, AI implementation requires the reproduction of content, and modern AI systems record the relationships between words in ways that can preserve and even reproduce the original work.
This phenomenon, known as “memorization,” means the storage of these copies can ultimately lead to the intact retrieval of portions of works, or in some cases, works in their entirety. There have been numerous examples of AI systems reproducing copyrighted work verbatim or nearly verbatim. The more unique the content, the more likely this reproduction becomes. ChatGPT 3.5, for instance, has been observed to regurgitate Dr. Seuss’s writing when prompted with “Oh, the places you’ll go.”
From a copyright and licensing perspective, the critical point here is that content fed into AI models can potentially be reproduced when the right prompts are used.
Implementation Challenges with Enterprise AI
Seventy-five percent of pharmaceutical executives cite generative AI as a C-suite or board priority, adding to the urgency organizations feel when solving the challenges blocking their implementation of effective AI workflows. These challenges go far beyond the issue of selecting the right AI workflow model, and they are more imperative in research and development organizations where specialized domain knowledge is critical.
A recent McKinsey study revealed that selecting the right AI model accounts for only about 15% of a typical AI project’s effort, contradicting the assumption that generative AI drops from the sky and immediately delivers value on its own. Most work involves adapting models to company-specific knowledge bases or tailoring them to particular use cases.
When examining AI adoption in enterprise settings, two categories emerge: training (pre-training foundation models, fine-tuning) and end-user workflows (content summarization, document interrogation). The second category is more prevalent in R&D spaces like life sciences, pharmaceuticals, and chemicals, where individuals use AI systems to help automate everyday tasks involving large amounts of content.
These end-user workflows typically involve activities like summarizing articles, extracting insights from documents more quickly, creating visualizations of content sets, or translating content. Despite enterprise-wide directives and some successes in less specialized use cases, organizations implementing AI initiatives in R&D environments consistently face the following challenges:
- Procuring content for fine-tuning or retrieval-augmented generation workflows, especially domain-relevant external content like peer-reviewed scientific literature
- Ensuring content is properly licensed for intended AI uses, particularly when subscription agreements rarely include secondary rights for AI activities
- Validating information quality throughout data pipelines to prevent the “garbage in, garbage out” problem
- Managing integration complexity across multiple content providers without creating an integration burden through heterogeneous systems
Organizations attempting to move beyond basic implementations into more specialized and impactful AI workflows must address these challenges to achieve long-term success.
System Requirements for Successful AI Implementation
A successful AI workflow hinges on the ability to model and resolve rights, access domain-relevant content, normalize and enrich data, and track data quality over time.
A proper rights management system must handle both standard license provisions and organization-specific rights negotiated with publishers and vendors. As published literature changes hands and licensing agreements vary in their terms, the system needs robust rights resolution capabilities. This means providing a single, clear answer to the question: “Can I use this content for my intended AI purpose?” based on the most permissive rights available.
From a practical perspective, organizations need rights-lookup APIs that can serve as decision points in data pipelines, determining whether to proceed with AI uses based on rights availability. Bulk lookup features can further streamline integration by managing the logic of large volume lookups.
Content normalization requires standardizing metadata elements like author names and affiliations, which can be especially messy in scientific data feeds. This normalization should be accompanied by enrichment—adding valuable identifiers and tags that improve findability and usability. For example, in biomedical literature, enrichment might include adding PubMed IDs and MeSH descriptors.
Measuring quality across dimensions of accuracy, completeness, consistency, currency, and redundancy helps build the strongest foundation for AI systems. Accuracy measures how faithfully records can represent real-world entities, and completeness evaluates whether records contain all expected fields. Consistency examines coherence both internally and across fields, currency indicates timeliness, and redundancy assessment helps identify undue bias in the corpus.
Consider a literature review workflow: when a researcher submits a question, the AI translates it into a proper search query, acquires full-text content (where properly licensed), and produces comprehensive answers with supporting evidence. The response typically includes both a narrative answer and deeper-level data that allows human validation. Without proper rights management and content procurement, this workflow would be incomplete or potentially infringing.
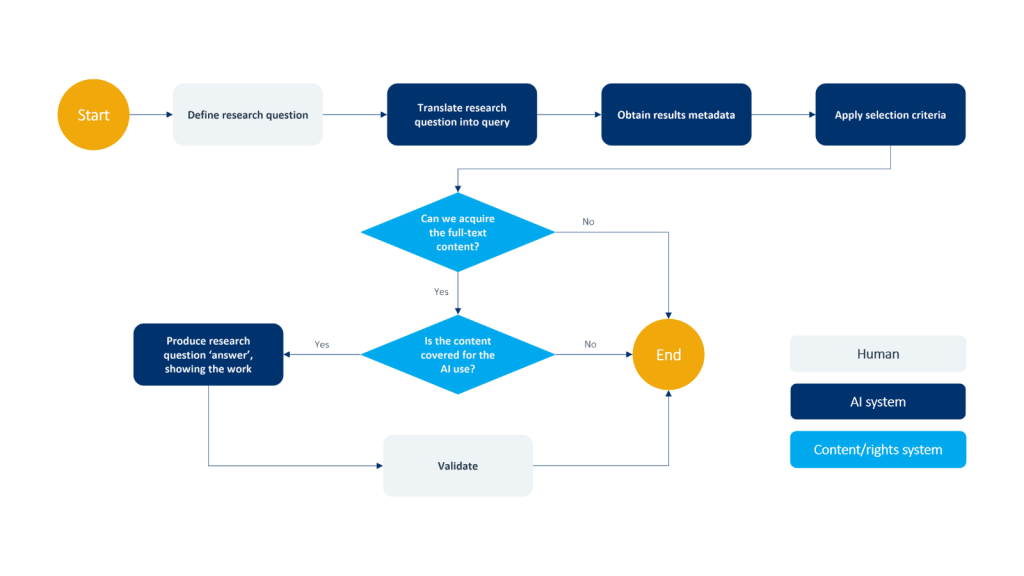
Creating a Path Forward with Rights-Enabled AI Workflows
Successful AI workflows require more than just selecting and implementing a model. Domain-specific tailoring is essential, and the organizations that effectively manage content procurement and copyright considerations will create significantly more valuable AI implementations.
CCC (Copyright Clearance Center) is working to help organizations address AI implementation challenges by incorporating rights for internal AI uses into its copyright license offerings. Rather than focusing on specific use cases or technologies (which quickly become outdated), CCC has created flexible licenses with reasonable limitations that cover activities like content analysis, model training, data processing, and content summarization.
These licenses apply only to lawfully obtained content, preserving the value of subscriptions while enabling AI innovation.
As organizations develop their AI strategy, they must remember that using high-quality, rights-cleared content will be a key differentiator in the effectiveness of their AI workflows. Those who solve these challenges now will be well-positioned to turn their proof-of-concepts into reality and embed them into real business workflows.